This gallery consists of samples taken from my design studios (Learnt Smart) conducted at the 2017 (7–15 July) AA Shanghai Visiting School (Architectural Association School of Architecture, London). An 11-day long summer workshop held at the China 3D Print Museum. A series of experiments was carried out to investigate the deep learning techniques of neural style transfer at the scale and in the context of architecture and urbanism.
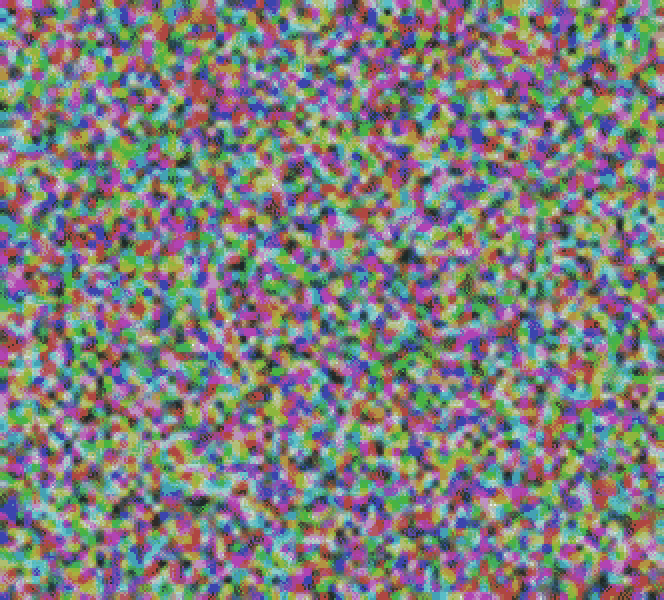
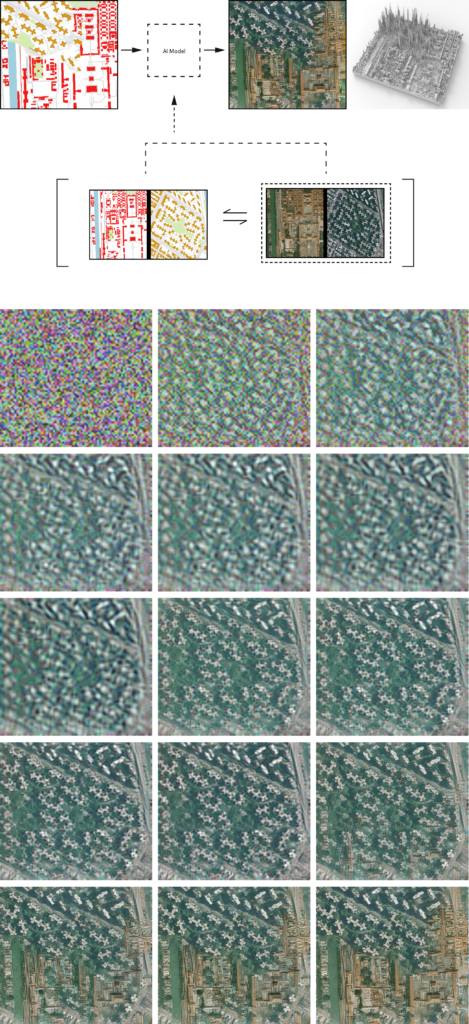
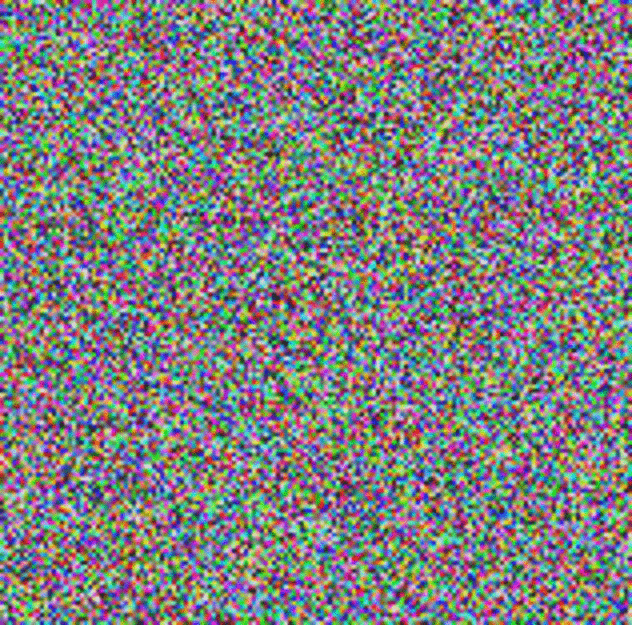
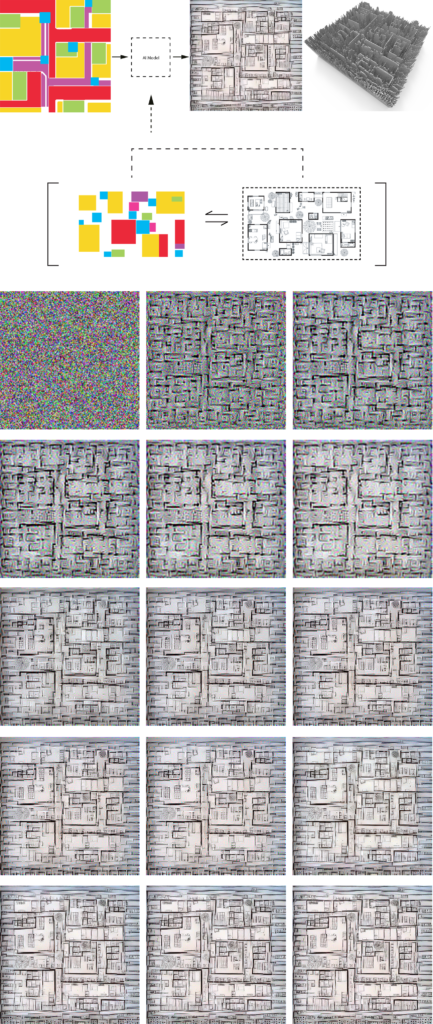
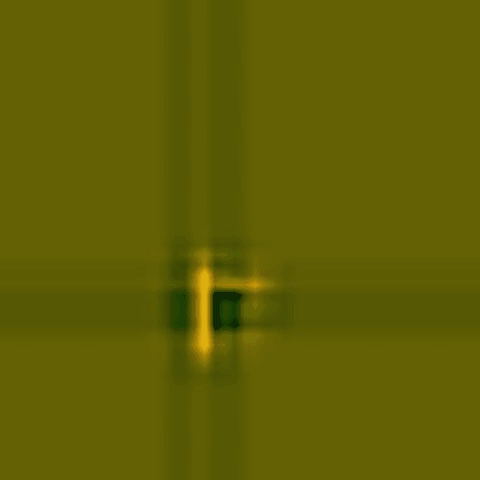
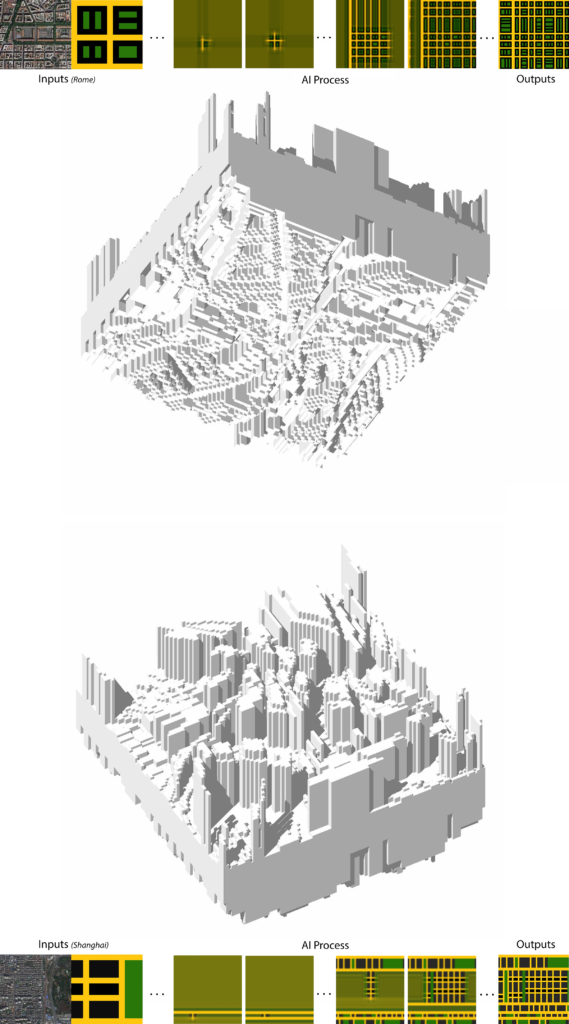
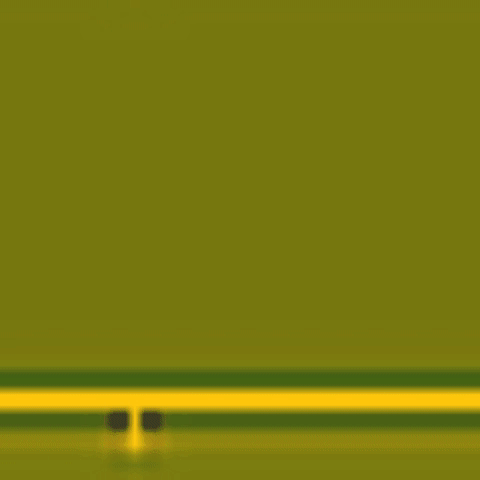
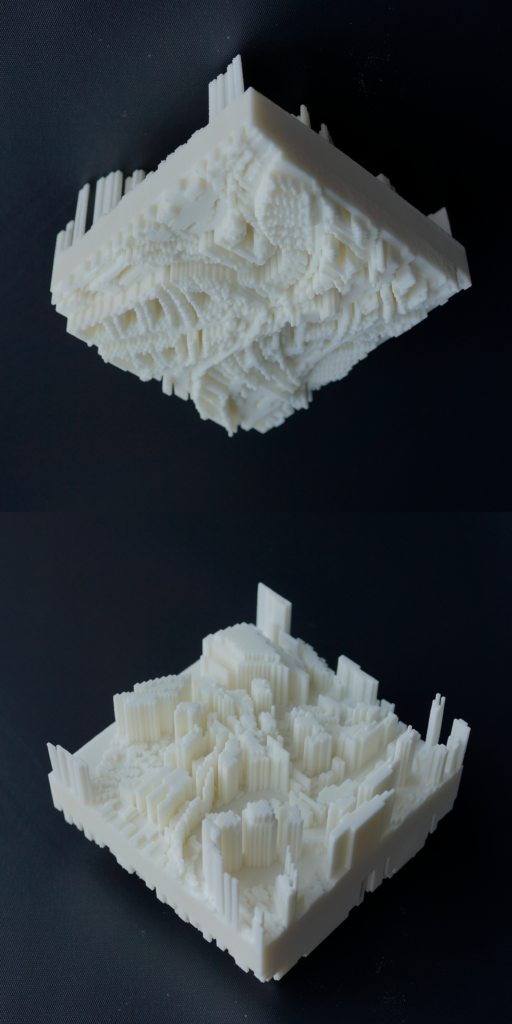